Deep Radiomics-based Fusion Model Predicting Bevacizumab Treatment Response and Outcome in Patients With Colorectal Liver Metastases
![]() |
The safety and scientific validity of this study is the responsibility of the study sponsor and investigators. Listing a study does not mean it has been evaluated by the U.S. Federal Government. Read our disclaimer for details. |
ClinicalTrials.gov Identifier: NCT06023173 |
Recruitment Status :
Completed
First Posted : September 5, 2023
Last Update Posted : September 14, 2023
|
- Study Details
- Tabular View
- No Results Posted
- Disclaimer
- How to Read a Study Record
Condition or disease | Intervention/treatment |
---|---|
The Patients With CRLM Who Benefit More From Bevacizumab | Diagnostic Test: Deep radiomics-based fusion model |
Study Type : | Observational |
Actual Enrollment : | 307 participants |
Observational Model: | Cohort |
Time Perspective: | Retrospective |
Official Title: | Deep Radiomics-based Fusion Model Predicting Bevacizumab Treatment Response and Outcome in Patients With Colorectal Liver Metastases: a Multicenter Cohort Study |
Actual Study Start Date : | October 1, 2013 |
Actual Primary Completion Date : | January 1, 2023 |
Actual Study Completion Date : | January 1, 2023 |
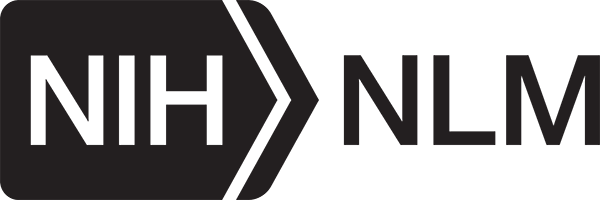
Group/Cohort | Intervention/treatment |
---|---|
Training Cohort
This cohort was derived from Arm A (treated with FOLFOX + bevacizumab) of the BECOME studyand was used for model construction.
|
Diagnostic Test: Deep radiomics-based fusion model
This multi-modal deep radiomics model, using PET/CT, clinical and histopathological data, was able to identify patients with bevacizumab-sensitive CRLM, providing a favorable approach for precise patient treatment.
Other Name: deep learning model |
Negative Validation Cohort
The cohort was derived from Arm B (treated with FOLFOX) of the BECOME study , which demonstrated that the model specifically predicted the efficacy of bevacizumab.
|
Diagnostic Test: Deep radiomics-based fusion model
This multi-modal deep radiomics model, using PET/CT, clinical and histopathological data, was able to identify patients with bevacizumab-sensitive CRLM, providing a favorable approach for precise patient treatment.
Other Name: deep learning model |
Internal Validation Cohort
The cohort was derived from an independent Zhongshan Hospital cohort with the same treatment team and imaging instrumentation as the BECOME study, differing only in patient period, and was used for internal validation of the model.
|
Diagnostic Test: Deep radiomics-based fusion model
This multi-modal deep radiomics model, using PET/CT, clinical and histopathological data, was able to identify patients with bevacizumab-sensitive CRLM, providing a favorable approach for precise patient treatment.
Other Name: deep learning model |
External Validation Cohort
The cohort was obtained from the Zhongshan Hospital - Xiamenand the First Affiliated Hospital of Wenzhou Medical University for external validation of the model.
|
Diagnostic Test: Deep radiomics-based fusion model
This multi-modal deep radiomics model, using PET/CT, clinical and histopathological data, was able to identify patients with bevacizumab-sensitive CRLM, providing a favorable approach for precise patient treatment.
Other Name: deep learning model |
- ORR [ Time Frame: 2013.10.1-2023.1.1 ]Objective response rate of patients with colorectal cancer liver metastases who treated with FOLFOX+bevacizumab/FOLFOX
- PFS [ Time Frame: 2013.10.1-2023.1.1 ]Progression-free survival of patients with colorectal cancer liver metastases who treated with FOLFOX+bevacizumab/FOLFOX
- OS [ Time Frame: 2013.10.1-2023.1.1 ]Overall survival of patients with colorectal cancer liver metastases who treated with FOLFOX+bevacizumab/FOLFOX
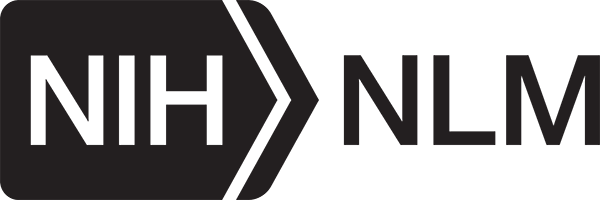
Choosing to participate in a study is an important personal decision. Talk with your doctor and family members or friends about deciding to join a study. To learn more about this study, you or your doctor may contact the study research staff using the contacts provided below. For general information, Learn About Clinical Studies.
Ages Eligible for Study: | 18 Years to 75 Years (Adult, Older Adult) |
Sexes Eligible for Study: | All |
Accepts Healthy Volunteers: | No |
Sampling Method: | Non-Probability Sample |
Inclusion Criteria:
- Age ≥ 18 years and ≤75 years;
- Patients were histologically confirmed for colorectal adenocarcinoma with unresectable liver-limited or liver-dominant metastases
- PET/CT at baseline were available
- First line treated with FOLFOX+ bevacizumab.
Exclusion Criteria:
- Resectable liver metastases;
- Wide-type KRAS/NRAS;
- No measurable liver metastasis;
- No efficacy assessment;
- No follow-up information.
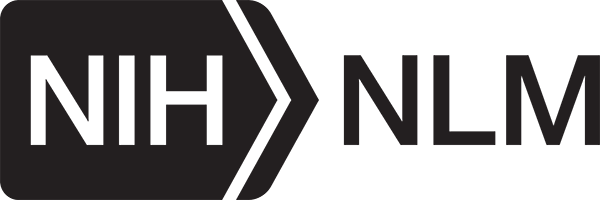
To learn more about this study, you or your doctor may contact the study research staff using the contact information provided by the sponsor.
Please refer to this study by its ClinicalTrials.gov identifier (NCT number): NCT06023173
China | |
Department of General Surgery, Zhongshan Hospital, Fudan University | |
Shanghai, China |
Principal Investigator: | Jianmin Xu, MD | Fudan University |
Responsible Party: | Xu jianmin, Head of Colorectal Surgery, Fudan University |
ClinicalTrials.gov Identifier: | NCT06023173 |
Other Study ID Numbers: |
DERBY |
First Posted: | September 5, 2023 Key Record Dates |
Last Update Posted: | September 14, 2023 |
Last Verified: | August 2023 |
Individual Participant Data (IPD) Sharing Statement: | |
Plan to Share IPD: | No |
Studies a U.S. FDA-regulated Drug Product: | No |
Studies a U.S. FDA-regulated Device Product: | No |
Neoplasm Metastasis Neoplastic Processes Neoplasms Pathologic Processes |