Construction of Early Warning Model for Pulmonary Complications Risk of Surgical Patients Based on Multimodal Data Fusion
![]() |
The safety and scientific validity of this study is the responsibility of the study sponsor and investigators. Listing a study does not mean it has been evaluated by the U.S. Federal Government. Know the risks and potential benefits of clinical studies and talk to your health care provider before participating. Read our disclaimer for details. |
ClinicalTrials.gov Identifier: NCT06057688 |
Recruitment Status :
Recruiting
First Posted : September 28, 2023
Last Update Posted : September 28, 2023
|
- Study Details
- Tabular View
- No Results Posted
- Disclaimer
- How to Read a Study Record
The goal of this observational study is to establish an intelligent early warning system for acute and critical complications of the respiratory system such as pulmonary embolism and respiratory failure. Based on the electronic case database of the biomedical big data research center and the clinical real-world vital signs big data collected by wearable devices, the hybrid model architecture with multi-channel gated circulation unit neural network and deep neural network as the core is adopted, Mining the time series trends of multiple vital signs and their linkage change characteristics, integrating the structural nursing observation, laboratory examination and other multimodal clinical information to establish a prediction model, so as to improve patient safety, and lay the foundation for the later establishment of a higher-level and more comprehensive artificial intelligence clinical nursing decision support system.
Issues addressed in this study
- The big data of vital signs of patients collected in real-time by wearable devices were used to explore the internal relationship between the change trend of vital signs and postoperative complications (mainly including infection complications, respiratory failure, pulmonary embolism, cardiac arrest). Supplemented with necessary nursing observation, laboratory examination and other information, and use machine learning technology to build a prediction model of postoperative complications.
- Develop the prediction model into software to provide auxiliary decision support for clinical medical staff, and lay the foundation for the later establishment of a higher-level and more comprehensive AI clinical decision support system.
Condition or disease |
---|
Pulmonary Embolism Respiratory Failure Infection Complication Cardiac Arrest |
The project prospectively collected the clinical information of patients in general surgery (gastrointestinal surgery, biliary surgery, pancreatic surgery), noninvasively monitored the patient's temperature, heart rate, ECG, respiratory rate through the body surface with wireless vital signs sensor, guided the patient to wear correctly from the first day of admission, and continuously monitored the patient's preoperative, intraoperative and postoperative vital signs throughout the whole process until leave hospital,The traditional "point" vital signs monitoring will be updated to continuous "line" monitoring, returning to the real world of patients' vital signs. The vital signs data of patients are continuously collected, transmitted in real-time and stored in the local central workstation of the ward, and the researchers of the project are specially assigned to be responsible for data export, storage and analysis. In view of the lack of early warning means for acute and critical complications in surgical ward, wearable devices with verified accuracy were used to collect continuous vital signs big data, fully mining the internal relationship between the change trend of patients' multiple vital signs parameters and complications, and establishing an intelligent risk warning model for perioperative complications of surgical patients, It lays the foundation for the establishment of a higher-level real-time patient risk warning and clinical decision support system, so as to improve the perioperative safety of patients and promote the penetration of artificial intelligence in clinical medicine and nursing.
Participants will use the Clavien Dindo grading criteria to determine the severity of complications according to the Cohort study method. According to whether the patient has experienced complications and the type of complications, the patient is divided into different subgroups and the patient outcome is manually calibrated. Mining the change trend of patient's vital signs over time, Convolutional neural network is used to extract morphological features from the continuous vital sign curves of the past 24 hours, and two-way short-term memory neural network is used to extract temporal features to obtain two sets of feature vectors. All data are feature fused, and sparse features are specially processed, and then sent to another weighted recurrent neural network to establish a complication prediction model, and predict the patient's Respiratory failure risk level, ICU risk level, and death risk level in the next 24 hours. Continuously revise the algorithm and set alarm threshold logic based on personal baseline data. Compare the predictive power of this model with the predictive power obtained from the national warning score NEWS, and determine the sensitivity, specificity, false positive rate, false negative rate, positive predictive value, and negative predictive value of the two in predicting patient outcomes.
Study Type : | Observational |
Estimated Enrollment : | 1770 participants |
Observational Model: | Cohort |
Time Perspective: | Prospective |
Official Title: | (Professor Gong Renrong) Ward Matron |
Actual Study Start Date : | August 1, 2023 |
Estimated Primary Completion Date : | October 1, 2024 |
Estimated Study Completion Date : | December 31, 2024 |
- Unplanned ICU admission [ Time Frame: During the whole hospitalization, the average was 7 days ]The patient was unplanned admitted to ICU due to pulmonary complications after operation
- Death [ Time Frame: During the whole hospitalization, the average was 7 days ]The patient died after surgery due to pulmonary complications
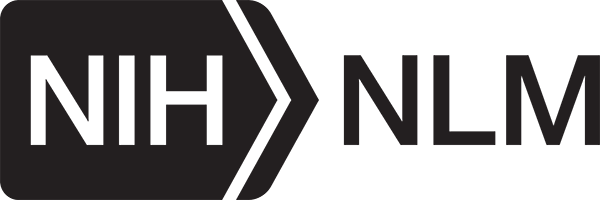
Choosing to participate in a study is an important personal decision. Talk with your doctor and family members or friends about deciding to join a study. To learn more about this study, you or your doctor may contact the study research staff using the contacts provided below. For general information, Learn About Clinical Studies.
Ages Eligible for Study: | 14 Years to 90 Years (Child, Adult, Older Adult) |
Sexes Eligible for Study: | All |
Accepts Healthy Volunteers: | Yes |
Sampling Method: | Probability Sample |
Inclusion Criteria:
- aged from 14 to 90 years; ② Patients undergoing surgery under general anesthesia; ③ Informed consent to participate in this study
Exclusion Criteria:
- The operation duration is less than 1 hour; ② Patients who cannot wear sensors for vital signs monitoring due to local skin abnormalities; ③ Combined with bilateral axillary surgery; ④ Incomplete bilateral axillary skin
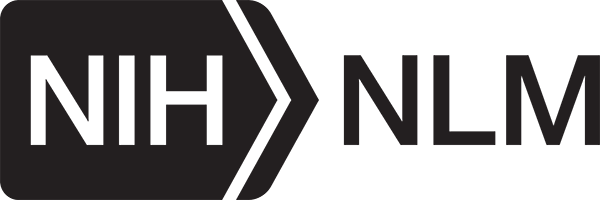
To learn more about this study, you or your doctor may contact the study research staff using the contact information provided by the sponsor.
Please refer to this study by its ClinicalTrials.gov identifier (NCT number): NCT06057688
Contact: GONG professor | +862885421887 | gongrenrong@wchscu.cn |
China, Sichuan | |
West China Hospital, Sichuan University. | Recruiting |
Chengdu, Sichuan, China, 610041 | |
Contact: 翠芳 曾 13518109099 zengcuifang007@163.com |
Study Director: | GONG professor | West China Hospital |
Responsible Party: | Renrong Gong, Prof.Gongrenrong, West China Hospital |
ClinicalTrials.gov Identifier: | NCT06057688 |
Other Study ID Numbers: |
ChiCTR2300072424 |
First Posted: | September 28, 2023 Key Record Dates |
Last Update Posted: | September 28, 2023 |
Last Verified: | September 2023 |
Studies a U.S. FDA-regulated Drug Product: | No |
Studies a U.S. FDA-regulated Device Product: | No |
Respiratory Insufficiency Pulmonary Embolism Embolism Respiration Disorders Respiratory Tract Diseases |
Cardiovascular Diseases Embolism and Thrombosis Vascular Diseases Lung Diseases |